Plenary Keynote Speakers
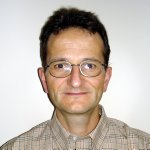
Recent Advances in Recommender Systems and Future Directions
Recommender systems are designed to identify the items that a user will like or find useful based on the user’s prior preferences and activities. These systems have become ubiquitous and are an essential tool for information filtering and (e-)commerce. Over the years, collaborative filtering, which derive these recommendations by leveraging past activities of groups of users, has emerged as the most prominent approach for solving this problem. Among the multitude of methods that have been developed, user- and item-based nearest-neighbor approaches are the simplest to understand and are easily extended to capture different user behavioral models and types of available information. However, in their classical forms, the performance of these methods is worse than latent-space approaches.
This talk presents an overview of recent methodological advances in developing nearest-neighbor-based recommender systems that have substantially improved their performance. The key components in these methods are: (i) the use of statistical learning to estimate from the data the desired user-user and item-item similarity matrices, (ii) the use of lower-dimensional representations to handle issues associated with sparsity, (iii) the combination of neighborhood and latent space models, and (iv) the direct incorporation of auxiliary information during model estimation. The talk will provide illustrative examples for these methods in the context of item-item nearest-neighbor methods for rating prediction and Top-N recommendation. In addition, the talk will present an overview of exciting new application areas of recommender systems along with the challenges and opportunities associated with them.
George Karypis is an ADC Chair of Digital Technology Professor at the Department of Computer Science & Engineering at the University of Minnesota, Twin Cities. His research interests spans the areas of data mining, high performance computing, information retrieval, collaborative filtering, bioinformatics, cheminformatics, and scientific computing. His research has resulted in the development of software libraries for serial and parallel graph partitioning (METIS and ParMETIS), hypergraph partitioning (hMETIS), for parallel Cholesky factorization (PSPASES), for collaborative filtering-based recommendation algorithms (SUGGEST), clustering high dimensional datasets (CLUTO), finding frequent patterns in diverse datasets (PAFI), and for protein secondary structure prediction (YASSPP). He has coauthored over 250 papers on these topics and two books (“Introduction to Protein Structure Prediction: Methods and Algorithms” (Wiley, 2010) and “Introduction to Parallel Computing” (Publ. Addison Wesley, 2003, 2nd edition)). In addition, he is serving on the program committees of many conferences and workshops on these topics, and on the editorial boards of the IEEE Transactions on Knowledge and Data Engineering, ACM Transactions on Knowledge Discovery from Data, Data Mining and Knowledge Discovery, Social Network Analysis and Data Mining Journal, International Journal of Data Mining and Bioinformatics, the journal on Current Proteomics, Advances in Bioinformatics, and Biomedicine and Biotechnology.
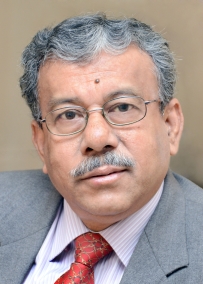
Granular Mining and Rough-Fuzzy Computing: Data to Knowledge and Big Data Issues
Pattern recognition and data mining in the framework of machine intelligence are explained. The role of rough sets in uncertainty handling and granular computing is highlighted. Relevance of its integration with fuzzy sets to result in a stronger paradigm for uncertainty handling is explained. Generalized rough sets, rough-fuzzy entropy, different f-information measures, and fuzzy granular social network (FGSN) model are described. FGSN handles the uncertainty arising from vaguely defined closeness or relations of the actors (nodes). Various measures towards this are stated.
Rough-fuzzy image entropy takes care of the fuzziness in boundary regions as well as the rough resemblance among nearby pixels and gray levels. Rough-fuzzy case generation with variable reduced dimension is useful for mining data sets with large dimension and size. Fuzzy granular model of social networks provides a generic platform for its analysis. Fuzzy-rough communities, detected thereby, are more significant when the degree of overlapping between communities increases. f-information measures quantify well the mutual information in efficient feature selection, and the conditional information in measuring the goodness of community structures in network mining. These characteristics are demonstrated for tasks like video tracking, social network analysis and gene/ microRNA selection. The role of different kinds of granules is illustrated as well as the concepts of fuzzy granular computing and granular fuzzy computing.
The talk concludes mentioning their relevance in handling Big data, the challenging issues and the future directions of research.
Sankar K. Pal is a Distinguished Scientist of the Indian Statistical Institute and its former Director. He is also a J.C. Bose Fellow of the Govt. of India and INAE Chair Professor. He founded the Machine Intelligence Unit and the Center for Soft Computing Research: A National Facility in the Institute in Calcutta. He received a Ph.D. in Radio Physics and Electronics from the University of Calcutta in 1979, and another Ph.D. in Electrical Engineering along with DIC from Imperial College, University of London in 1982.
Prof. Pal worked at the University of California, Berkeley and the University of Maryland, College Park in 1986-87; the NASA Johnson Space Center, Houston, Texas in 1990-92 & 1994; and in US Naval Research Laboratory, Washington DC in 2004. Since 1997 he has been a Distinguished Visitor of the IEEE Computer Society, for the Asia-Pacific Region, and held several visiting positions in Italy, Poland, Hong Kong and Australian universities.
He is a co-author of seventeen books and more than four hundred research publications in the areas of Pattern Recognition and Machine Learning, Image Processing, Data Mining and Web Intelligence, Soft Computing, Neural Nets, Genetic Algorithms, Fuzzy Sets, Rough Sets and Bioinformatics. He visited about forty countries as a Keynote/ Invited speaker.
He is a Fellow of the IEEE, the Academy of Sciences for the Developing World (TWAS), International Association for Pattern Recognition, International Association of Fuzzy Systems, and all the four National Academies for Science & Engineering in India. He serves(d) in the editorial boards of twenty-two international journals including several IEEE Transactions.
He has received the 1990 S.S. Bhatnagar Prize (the most coveted award for a scientist in India), 2013 Padma Shri (one of the highest civilian awards) by the President of India and many prestigious awards in India and abroad including the 1999 G.D. Birla Award, 1993 Jawaharlal Nehru Fellowship, 2000 Khwarizmi International Award from the President of Iran, 1993 NASA Tech Brief Award (USA), 1994 IEEE Trans. Neural Networks Outstanding Paper Award, and 2005-06 Indian Science Congress-P.C. Mahalanobis Birth Centenary Gold Medal from the Prime Minister of India for Lifetime Achievement.
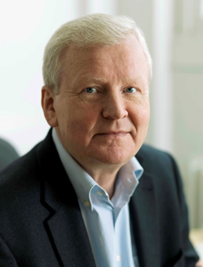
Constructive Learning of Preferences with Robust Ordinal Regression
The talk is devoted to preference learning in Multiple Criteria Decision Aiding. It is well
known that the dominance relation established in the set of alternatives (also called actions, objects,
solutions) is the only objective information that comes from a formulation of a multiple criteria decision
problem (ordinal classification, or ranking, or choice, with multiobjective optimization being a particular
instance). While dominance relation permits to eliminate many irrelevant (i.e., dominated) alternatives, it
does not compare completely all of them, resulting in a situation where many alternatives remain
incomparable. This situation may be addressed by taking into account preferences of a Decision Maker
(DM). Therefore, all decision-aiding methods require some preference information elicited from a DM or
a group of DMs. This information is used to build more or less explicit preference model, which is then
applied on a non-dominated set of alternatives to arrive at a recommendation (assignment of alternatives
to decision classes, or ranking of alternatives from the best to the worst, or the best choice) presented to
the DM. In practical decision aiding, the process composed of preference elicitation, preference modeling,
and DM’s analysis of a recommendation, loops until the DM accepts the recommendation or decides to
change the problem setting. Such an interactive process is called constructive preference learning.
I will focus on processing DM’s preference information concerning multiple criteria ranking and choice
problems. This information has the form of pairwise comparisons of selected alternatives. Research
indicates that such preference elicitation requires less cognitive effort from the DM than direct assessment
of preference model parameters (like criteria weights or trade-offs between conflicting criteria). I will
describe how to construct from this input information a preference model that reconstructs the pairwise
comparisons provided by the DM. In general, construction of such a model follows logical induction,
typical for learning from examples in AI. In case of utility function preference models, this induction
translates into ordinal regression. I will show inductive construction techniques for two kinds of
preference models: a set of utility (value) functions, and a set of “if…, then…” monotonic decision rules.
An important feature of these construction techniques is identification of all instances of the preference
model that are compatible with the input preference information – this permits to draw robust conclusions
regarding DM’s preferences when any of these models is applied on the considered set of alternatives.
These techniques are called Robust Ordinal Regression and Dominance-based Rough Set Approach.
I will also show how these induction techniques, and their corresponding models, can be embedded into
an interactive procedure of multiobjective optimization, particularly, in Evolutionary Multiobjective
Optimization (EMO), guiding the search towards the most preferred region of the Pareto-front.
Roman Słowiński is a Professor and Founding Chair of the Laboratory of Intelligent Decision Support Systems at the Institute of Computing Science, Poznań University of Technology in Poland. Since 2002 he is also Professor at the Systems Research Institute of the Polish Academy of Sciences in Warsaw. He is a full member of the Polish Academy of Sciences and, presently, elected president of the Poznań Branch of the Academy. He is also a member of Academia Europaea. In his research, he combines Operations Research and Computational Intelligence. Today Roman Słowiński is renown for his seminal research on using rough sets in decision analysis, and for his original contribution to preference modeling and learning in decision aiding. He is recipient of the EURO Gold Medal, and Doctor Honoris Causa of Polytechnic Faculty of Mons, University Paris Dauphine, and Technical University of Crete. In 2005 he received the Annual Prize of the Foundation for Polish Science - regarded as the highest scientific honor awarded in Poland. Since 1999, he is principal editor of the European Journal of Operational Research, a premier journal in Operations Research. He is coordinator of the EURO Working Group on Multiple Criteria Decision Aiding, and past president of the International Rough Set Society.
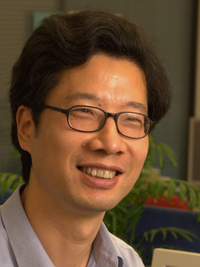
Ensemble Approaches in Learning
Designing a monolithic system for a large and complex learning task is hard.
Divide-and-conquer is a common strategy in tackling such large and complex
problems. Ensembles can be regarded an automatic approach towards automatic
divide-and-conquer. Many ensemble methods, including boosting, bagging,
negative correlation, etc., have been used in machine learning and data mining
for many years. This talk will describe three examples of ensemble methods,
i.e., multi-objective learning, online learning with concept drift, and
multi-class imbalance learning. Given the important role of diversity in
ensemble methods, some discussions and analyses will be given to gain a better
understanding of how and when diversity may help ensemble learning.
Some materials used in the talk were based on the following papers:
A Chandra and X. Yao, Ensemble learning using multi-objective evolutionary
algorithms, Journal of Mathematical Modelling and Algorithms, 5(4):417-445,
December 2006.
L. L. Minku and X. Yao, DDD: A New Ensemble Approach For Dealing With Concept
Drift, IEEE Transactions on Knowledge and Data Engineering, 24(4):619-633,
April 2012.
S. Wang and X. Yao, Multi-Class Imbalance Problems: Analysis and Potential
Solutions, IEEE Transactions on Systems, Man and Cybernetics, Part B,
42(4):1119-1130, August 2012.
Xin Yao is a Chair (Professor) of Computer Science and the Director of CERCIA (Centre of Excellence for Research in Computational Intelligence and Applications) at the University of Birmingham, UK. He is the President (2014-15) of IEEE Computational Intelligence Society (CIS). His work won the 2001 IEEE Donald G. Fink Prize Paper Award, 2010 IEEE Transactions on Evolutionary Computation Outstanding Paper Award, 2010 BT Gordon Radley Award for Best Author of Innovation (Finalist), 2011 IEEE Transactions on Neural Networks Outstanding Paper Award, and many other best paper awards. He won the prestigious Royal Society Wolfson Research Merit Award in 2012 and the 2013 IEEE CIS Evolutionary Computation Pioneer Award. He was the Editor-in-Chief (2003-08) of IEEE Transactions on Evolutionary Computation and is an Associate Editor or Editorial Member of more than ten other journals. His major research interests include evolutionary computation, ensemble learning, and their applications, especially in software engineering.